With news avoidance an ongoing problem for publishers, should news be covered from a more positive perspective? We know that engagement is given a boost when topics are covered through a mix of emotion-driven and action-driven needs, so we asked our data team to see how user need drivers relate to positive or negative words in articles.
From our Labs Team: this is what we know about negative news sentiment and user needs
Sept. 10, 2024 by Bojana Soro
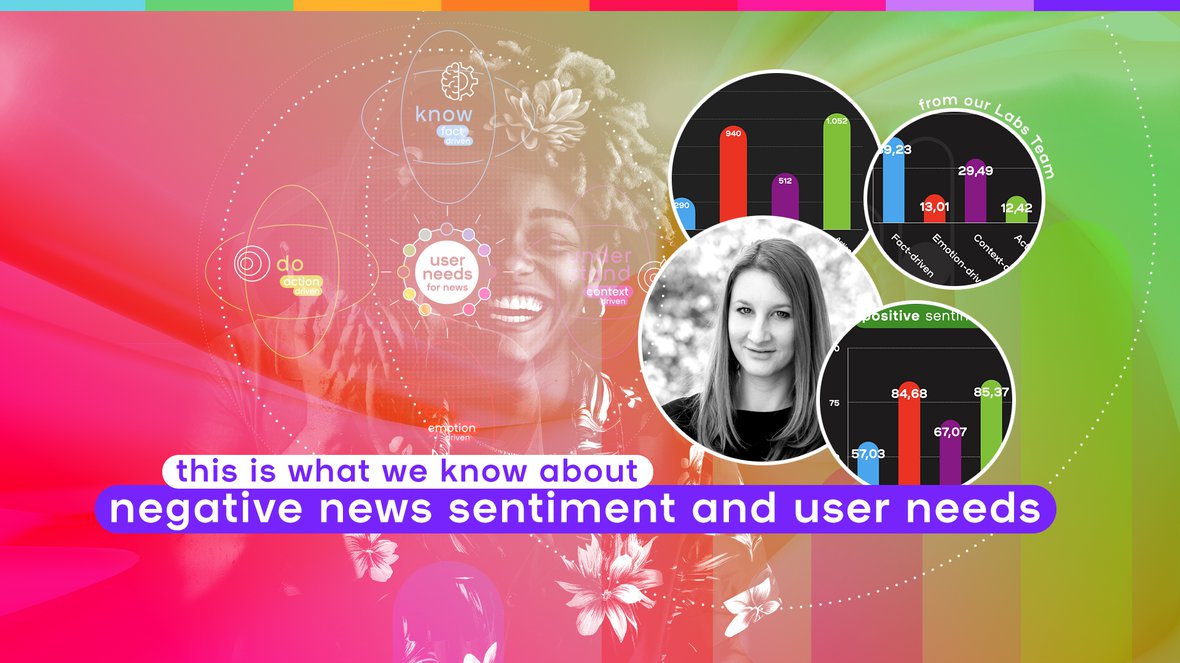
smartocto's senior data analyst, Bojana Soro, wrote this guest blog on the practical use of automated tagging with GenAIs.

Bojana Soro Senior Data Analyst
Recent analysis from Reuters Institute for the Study of Journalism focused on negative and positive sentiment in news. This may give editors pause over how to best frame stories: studies suggest that there has been an increase in negative headlines and articles in the two decades up to 2020, and the report shared comments such as that “the negative nature of the news itself makes [readers] feel anxious and powerless”.
These are comprehensive studies, and make for fascinating reading (do that here and here), but they don’t tell the whole story, and we were curious what happens when you throw user needs analysis into the mix. The resultant findings are below, authored by our Senior Data Analyst, Bojana Soro.
The question
Do certain user needs perspectives, e.g. writing predominantly fact-driven or emotion-driven articles, drive higher levels of negative sentiment in news?
The process
- Our research utilised a sample of almost 4,000 articles in English, all collected in 2023, with each news piece categorised into one of our four User Needs Model 2.0 model key categories: action, emotion, fact, and context.
- The news articles were collected via a commercial news aggregator API, spanning numerous and various sources.
- The dataset was carefully balanced, with approximately 1,000 articles in each category included in the analysis.
- We applied our in-house developed system for automatic tagging of user needs in news articles to score (0-100) each article on the fact-driven, context-driven, emotion-driven, and action-driven axes of the User Needs 2.0 model. The predominant User Needs axis for each article was taken to be the one with the maximum score for that article. For example, an article receiving a score of 40 on the fact-driven axis, 53 on the context-driven axis, 5 on the emotion-driven axis, and 2 on the action-driven axis, would be categorised as a context-driven article.
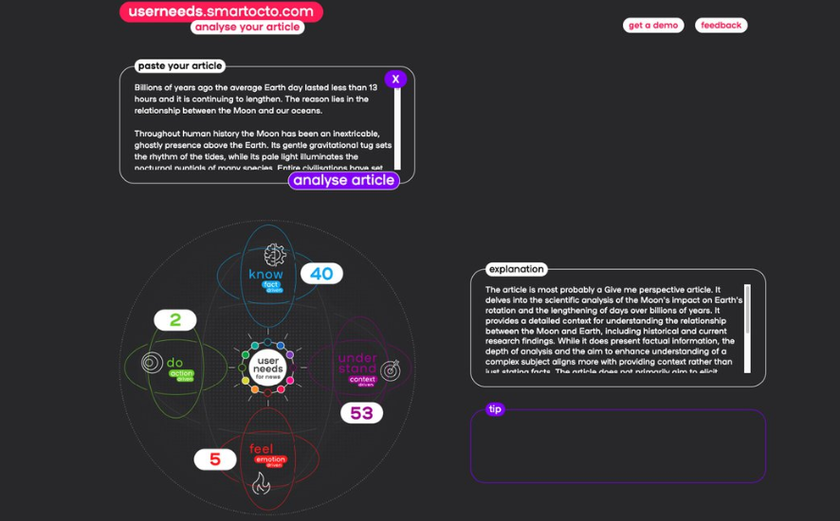
- We were able to tag a massive amount of articles, so we started with tens of thousands, and finally sampled 1,000 articles from each of the axes to balance out the sample. Having a balanced sample was important to us since we aimed to statistically compare the mean sentiment score in each category and draw conclusions from there.
A quick note on Sentiment Analysis
Sentiment analysis, a specialised area of natural language processing (NLP), enables companies to identify the emotional tone embedded within text, whether it’s positive, negative, or neutral. Sentiment analysis integrates data mining, machine learning (ML), artificial intelligence (AI), and computational linguistics to evaluate and classify emotions expressed in text (see here, for example). The process involves more than just detecting sentiment; it also measures the intensity of the sentiment and might attempt to identify the subject and the opinion holder within the text.
Several techniques are employed to achieve this.
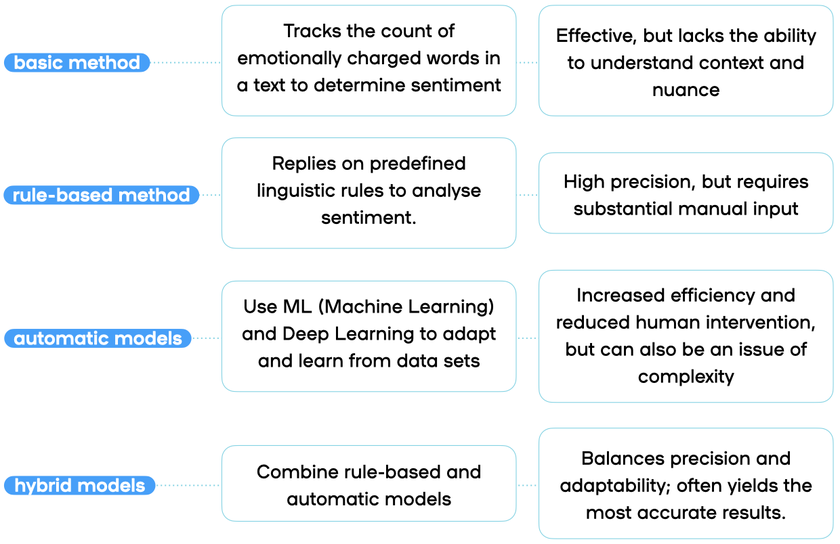
In this research study, we employed an advanced approach to sentiment analysis, based on generative AI. Our process begins by extracting all named entities within the text. In computational linguistics, a named entity refers to specific pieces of text that identify something or someone by name. These could be the names of people, places, organisations, or even dates and amounts of money. Essentially, the process picks out specific names or terms so it can understand who or what is being talked about. For instance, in the sentence "Paris is the capital of France," both "Paris" and "France" would be named entities since they are names of specific places. Similarly, in the sentence "Barack Obama served as the President of the United States", "Barack Obama" and "United States" are named entities as they refer to a specific person and a specific country.
Each identified entity is then assigned a sentiment score on a scale from -3 to 3, where negative values indicate a negative sentiment tone, zero represents a neutral tone, and positive values reflect a positive tone. The process of assigning sentiment scores to entities was done by taking into account the way in which every particular entity is treated in the news article at hand. We did not look at the sentiment of the headline alone, or the full text of the news piece as a whole, rather allowing that its author could have presented some entities in a positive light, while presenting some other entities in a more negative light. This method is effective because it takes the entire context of the text into account when determining the sentiment of particular entities mentioned. Finally, the overall sentiment of the text is calculated as the average of all entity sentiment scores within the text.
Sentiment in User Needs Model 2.0
We then asked how the sentiment of texts shifts depending on the dominant User Needs axis to which any particular news article in our study sample belongs to. We first categorised article sentiment scores into negative, neutral, and positive, and then counted the percent of articles falling into each of these three categories separately for the fact-driven, context-driven, emotion-driven, and action-driven articles. The chart below illustrates our result.
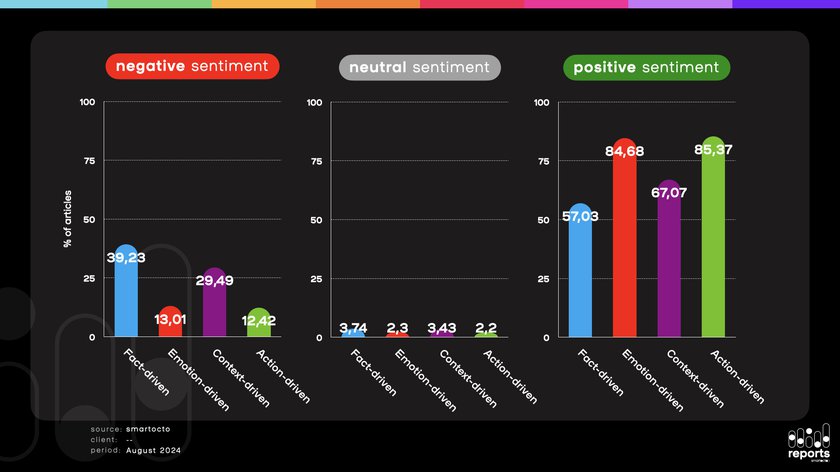
It’s clear that when examining the sentiment of news articles in this way, emotion-driven and action-driven axes have a lower percentage of negative content, while the opposite is true for fact-driven and context-driven articles in which negative content prevails. This leads us to a very important insight: namely, the shift towards more negative news is not driven equally by all user needs, with emotion-driven and action-driven articles balancing up to a certain level the negativity that is more present in hard news typically framed as fact-driven and context-driven stories.
In addition, research conducted as part of the smartocto User Needs Labs project has shown that increasing the number of articles in the emotion-driven and action-driven categories also boosts audience engagement. This type of content is particularly appealing to younger generations, as it offers them inspiring stories that motivate and encourage action, rather than focusing on darker topics.
We next compared the average sentiment score across all four User Needs Model 2.0 axes. The following chart represents the User Needs categories on the horizontal axis and the mean sentiment score on the vertical axis. The gap in mean sentiment score between emotion and action-driven articles on one hand, and fact and context-driven articles on the other hand, is striking.
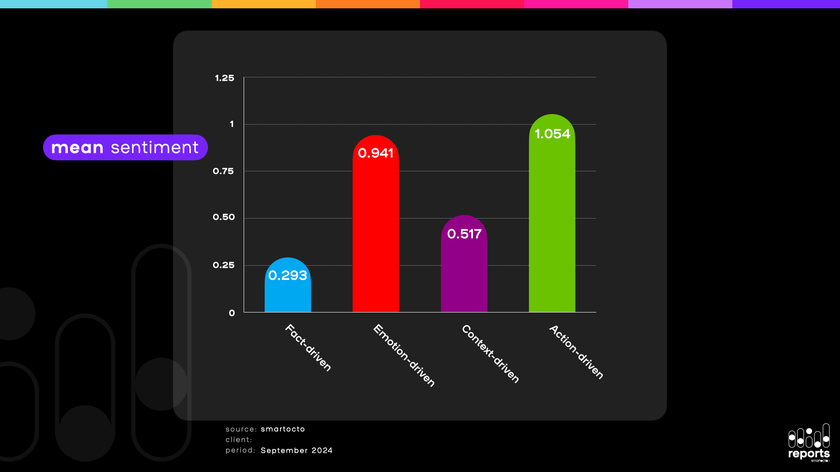
The results - based on a statistical model known as ANOVA (Analysis of Variance) - reveal that the average sentiment across all User Needs categories differs significantly. Follow-up statistical tests confirmed that all pairwise differences (e.g. fact-driven vs. context-driven, emotion-driven vs fact-driven, etc) were also significant. Interestingly enough, the highest positive average sentiment is found in the predominantly action-driven articles. Also, in spite of the fact that the typical hard news category of fact-driven articles received the lowest average sentiment score, that score is still positive (around 0.2 on a -3 to 3 scale, only slightly above neutral).
You might think that findings like the ones presented here directly imply that adopting a certain perspective from the User Needs Model 2.0 will automatically lead to producing news with a more positive sentiment. That is certainly not true. The results we see are undoubtedly, to a large extent, a consequence of the differences in the very content that the media typically cover in the fact-driven, context-driven, emotion-driven, and action-driven categories. If daily news reports, whose primary task is to keep the public well-informed, are filled with news about wars, regional conflicts, or economic inflation, it is clear that not much positive sentiment can be expected there. Perhaps emotion-driven and action-driven articles are simply the forms in which the media choose to cover some brighter topics.
But something else is also true: there is no obstacle to writing about the same event - regardless of how negative or positive it may be - from the perspective of the emotion-driven or action-driven axes of the model. As we noted in our User needs and AI: a deep dive in the data bunker blog post, the relationship between the sentiment of the text and its User Needs axis is complex:
The essence of the User Needs model is that practically any event can be written about from any axis of the model. You can start with a fact-driven article on some event, follow up by a contextual ‘Give me perspective’ type of story and then attempt at even higher audience engagement by publishing an emotion-driven story. That is why the question of how to computationally recognise which user needs an article satisfies cannot be resolved solely by analysing the meaning - the semantics - of the words and phrases in the text; it requires analysing how the author uses the language in the text, how it addresses different audiences, what its goal is, what effect it wants to achieve, and what reaction it aims to provoke.

Goran S. Milovanović Chief AI Officer
Wars? Earthquakes? Fake news? Dirty political campaigns? Reporting on these topics can hardly be done with a positive sentiment. However, if your follow-up news articles are focused on gathering personal testimonies, people's perceptions, reactions, or on connecting people in search of solutions to similar problems, you will be able to inject that missing positivity yourself. In bad times, give hope. Illustrate the case of someone who figured out how to avoid the negative consequences of the reality you are writing about. Point them to people in similar situations who might help. Show them how they can do something that spreads the light of more open, brighter colours than hard news.
In conclusion?
The main takeaway here is, then, not that emotion-driven and action-driven axes of the User Needs Model 2.0 somehow determine the higher positive sentiment of the news, but that they have a potential to do so when chained as follow-ups to fact and context-driven articles in an appropriate editorial strategy.
In relation to the result concerning the growing negativity of the news, which motivated our research, we have just one more thing to add. We have seen how the emotion-driven and action-driven categories exhibit more positive sentiment than other axes of the User Needs Model 2.0. Everything we have previously learned about this model has shown us that these are also the categories that lead to higher user engagement. Therefore, anyone in journalism today who believes that sensationalism and shocking readers with dark headlines and distressing topics can set their media apart from others is seriously mistaken.
you may also like...
7 ways to create more engaging content
Every company needs relevant and appealing content in order to engage your audience. And when you can engage your audience, your company will grow. In this blog, we wrote down 7 tips & tricks to create more engaging content.
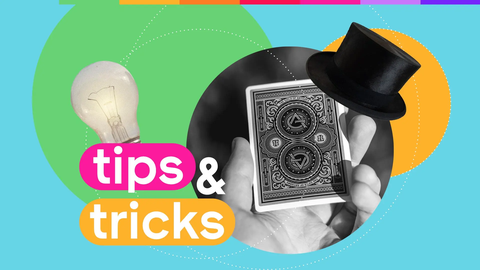
The added value of smart notifications
We're always talking about how smartocto is an actionable editorial analytics tool, but what does this mean? It's all about the smart notifications, explained right here!
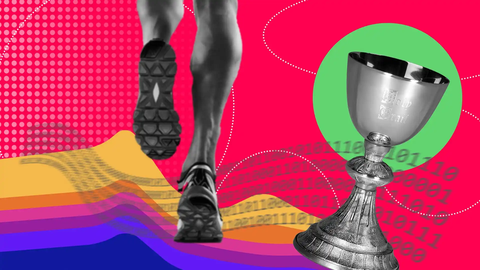